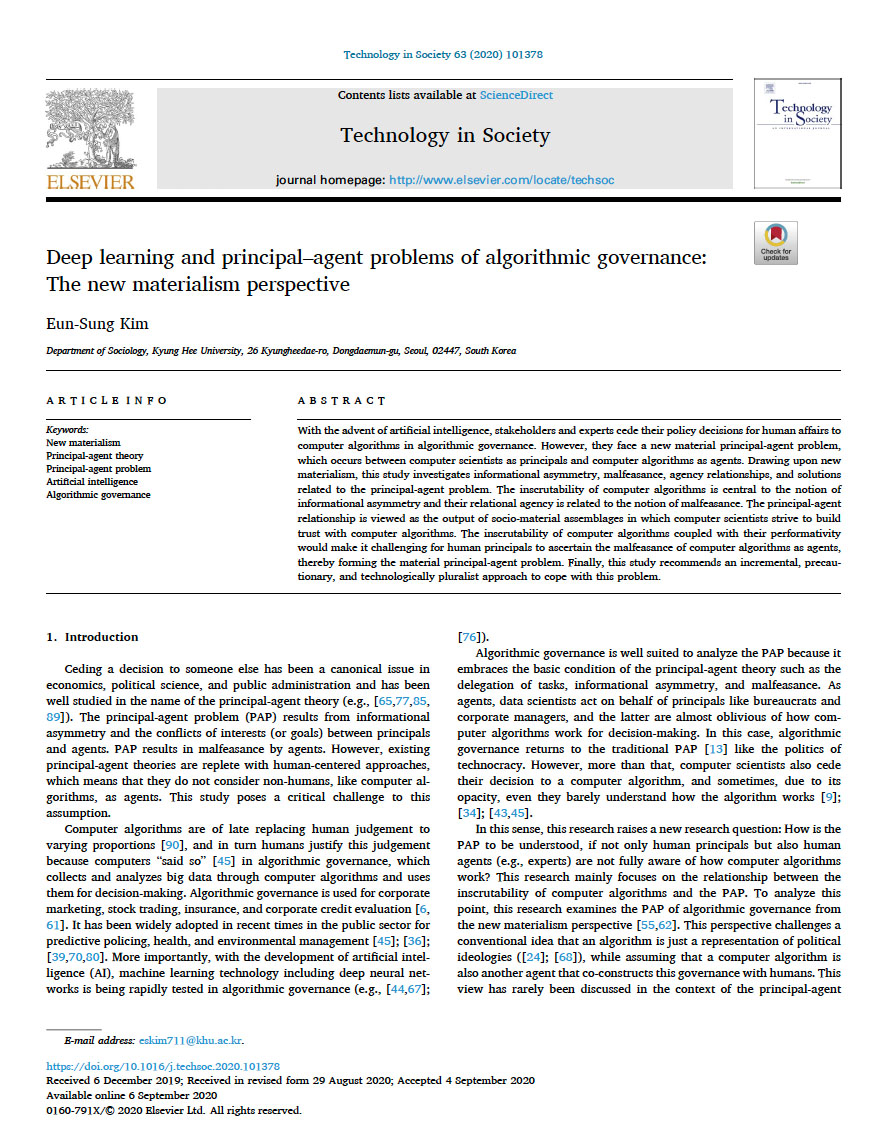
Title |
: Deep learning and principal-agent problems of algorithmic governance: the new materialism perspective |
Date |
: November, 2020 |
Journal title |
: Technology in Society |
Author |
: Kim, Eun-sung |
Contact us |
: csts@khu.ac.kr |
Abstract |
: With the advent of artificial intelligence, stakeholders and experts cede their policy decisions for human affairs to computer algorithms in algorithmic governance. However, they face a new material principal-agent problem, which occurs between computer scientists as principals and computer algorithms as agents. Drawing upon new materialism, this study investigates informational asymmetry, malfeasance, agency relationships, and solutions related to the principal-agent problem. The inscrutability of computer algorithms is central to the notion of informational asymmetry and their relational agency is related to the notion of malfeasance. The principal-agent relationship is viewed as the output of socio-material assemblages in which computer scientists strive to build trust with computer algorithms. The inscrutability of computer algorithms coupled with their performativity would make it challenging for human principals to ascertain the malfeasance of computer algorithms as agents, thereby forming the material principal-agent problem. Finally, this study recommends an incremental, precautionary, and technologically pluralist approach to cope with this problem. |
|